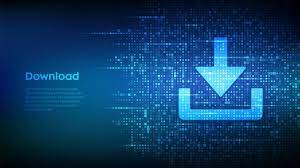

Library(scds) #- Annotate doublet using co-expression based doublet scoring: umi = cxds(umi) #- Annotate doublet using binary classification based doublet scoring: umi = bcds(umi) # train-error:0.066402+0.005931 test-error:0.103638+0.025177 SVI Bioinformatics and Cellular Genomics.19.2 External RNA Control Consortium (ERCC).17 “Ideal” scRNAseq pipeline (as of Oct 2019).16.14 Further subdivisions within cell types.16.13 Assigning cell type identity to clusters.16.12 Finding differentially expressed genes (cluster biomarkers).16.10 Run Non-linear dimensional reduction (tSNE).16.8 Determine statistically significant principal components.16.7 Perform linear dimensional reduction.16.6 Scaling the data and removing unwanted sources of variation.16.5 Detection of variable genes across the single cells.16.3 QC and selecting cells for further analysis.15 Integrating single-cell ’omics datasets.14.3 Projecting cells onto annotated cell-types (scmap).14 Comparing and combining scRNA-seq datasets.
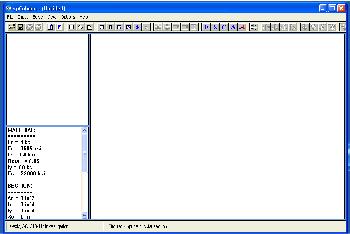
12.1.5 Models of single-cell RNA-seq data.12.1.4 Benchmarking of DE methods for scRNA-seq data.12 Differential Expression (DE) analysis.11.3.1 GAM general additive model for identifying temporally expressed genes.10.3 An alternative to clustering: Automatic cell annotation.Graph-based clustering (segerstolpe dataset) 9.2.1 Slalom: Interpretable latent spaces.9.2 Matrix factorization and factor analysis.9.1.2 tSNE: t-Distributed Stochastic Neighbor Embedding.9.1.1 PCA: Principal component analysis.8.1 Challenge: Handling sparsity in single-cell RNA sequencing.7.8.1 Identifying Genes vs a Null Model.
PCA COLUMN 64 BIT 4SHARED HOW TO
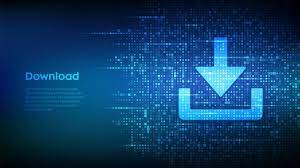